Characterizing home activities including both individual and family activities is important for studies of health, sociology, and home economics. This project develops a system that leverages pervasive smart devices for recognizing important home activities. The system is based on Federated Learning technologies that enable smart devices to collaboratively improve the accuracy of human activity sensing without sharing any sensor data, thus preserving user privacy. We address the imbalance data distribution issue in federated learning and explore the similarity of users to improve the activity recognition accuracy and reduce the energy consumptions on smart devices.
A similarity-aware federated learning system towards imbalanced data distribution. Nodes will first rectify the imbalanced local data distribution by themselves. The server first clusters users according to the uploaded machine learning models rather than raw sensor data, and further optimize the accuracy and communication performance based on the cluster relationship
Uniqueness and Competitive Advantages:
- The system is applicable to several sensory modalities
- Significant accuracy improvement towards rare activities
- Great accuracy improvement on various activity recognition tasks
- Less communication latency and energy consumptions on small smart devices
- Robust system performance under dynamic network conditions
- Can be used for behaviour analysis for Alzheimer’s Disease and childhood obesity analysis
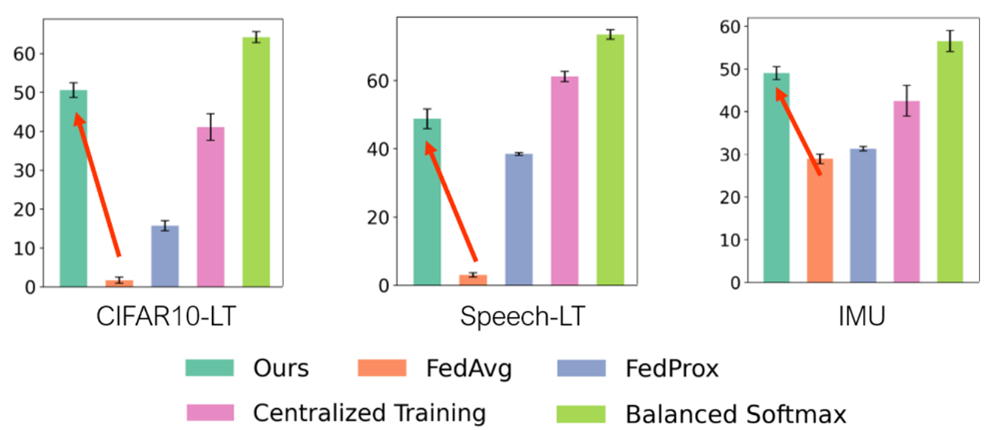
Mean accuracy and communication cost of different approaches with the number of nodes increasing. The left figure shows that our proposed system can improve the accuracy for most of nodes; the right figure shows the system can significantly reduce the communication time while maintaining the accuracy performance