Lung-Sight
Automated detection of pulmonary nodules via deep neural networks
Lung cancer has been the leading cause of cancer deaths worldwide. Visual inspection of lung tumors through medical imaging is one tedious and time-consuming task for radiologists and doctors. We propose a novel deep-learning-based framework with 3D convolutional networks for screening of primary lung cancer by automatically detecting pulmonary nodules from low-dose CT (Computed Tomography) images. Time and cost for screening will be significantly reduced and hence it will be beneficial to early diagnosis and treatment of lung cancer.
- Our proposed framework consists of two stages: 1) candidate screening, and 2) false positive reduction.
- Different from previous standard deep learning based methods, we try to tackle the severe hard/easy sample imbalance problem in medical datasets and explore the benefits of localized annotations to regularize the learning.
Breast-Histo Sight
Using deep learning technology to automatically detect mitosis in breast cancer histology images
The detection of lymph node metastasis gives an important aggressiveness indication of the invasive breast cancer. However, visual inspection relying on pathologists is time-consuming and expensive. To enhance the diagnosis of breast cancer, we propose a fast and robust method to detect mitosis by designing a novel deep cascaded convolutional neural network.
The deep cascaded neural network is composed of two components:
- First, by leveraging the fully convolutional network, a coarse retrieval model will identify and locate the candidates of mitosis while preserving a high sensitivity.
- Then, based on these candidates, a fine discrimination model is developed to further single out lymph node metastasis.
Cervix-Sight
Screening of cervical cancer by automatically detecting suspected cell malformation in digitalized cervical smear
Cervical cancer is one of the most common cancers in women. Screening is the only way to detect and cure cervical cancer at the early stage. Therefore, early screening to detect precancerous cervix is the key to preventing and treating cervical cancer. Based on the convolution neural network of deep learning technology for Thinprep Cytologic Test (TCT) detection of cervical cancer.
- Cervical cancer is screened by automatically detecting suspected cell malformation with subtypes classified in digitalized cervical smear.
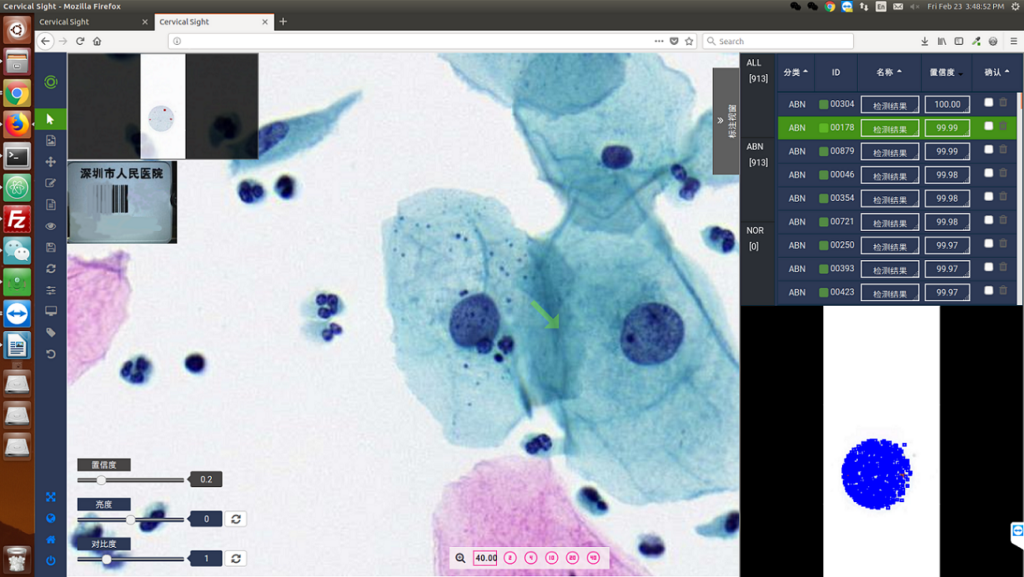
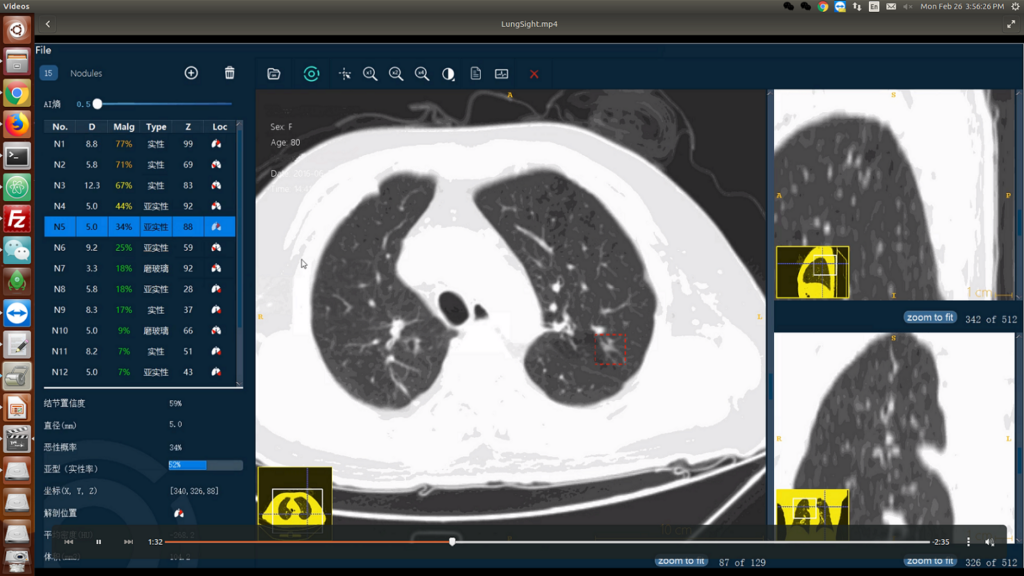
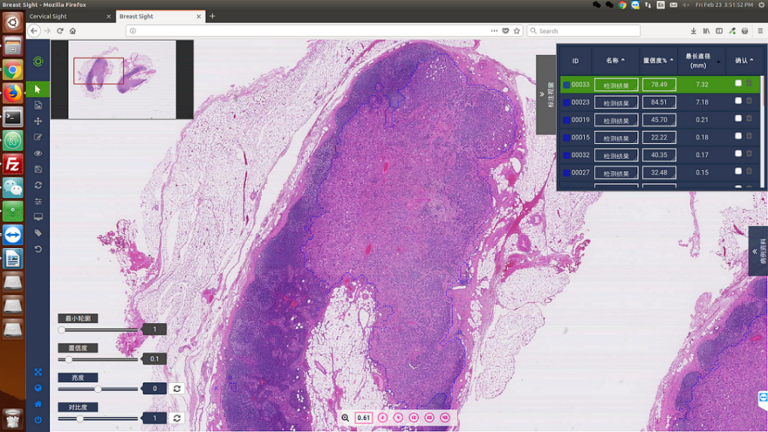