Early identification of people at risk of developing Alzheimer’s Disease (AD) and timely intervention to slow the onset and progression of AD are crucial.
In this project, we will build an indoor monitoring system that deploys different types of sensors and collect the corresponding data to develop a multi-modal fusion algorithm for daily activities and behavior detection. Besides, we will develop a new federated learning framework, which not only ensures the real-time of related algorithms but also protects users’ private data. After that, we will predict and identify individuals who are at a higher chance of developing dementia and provide high-quality feedback to the users.
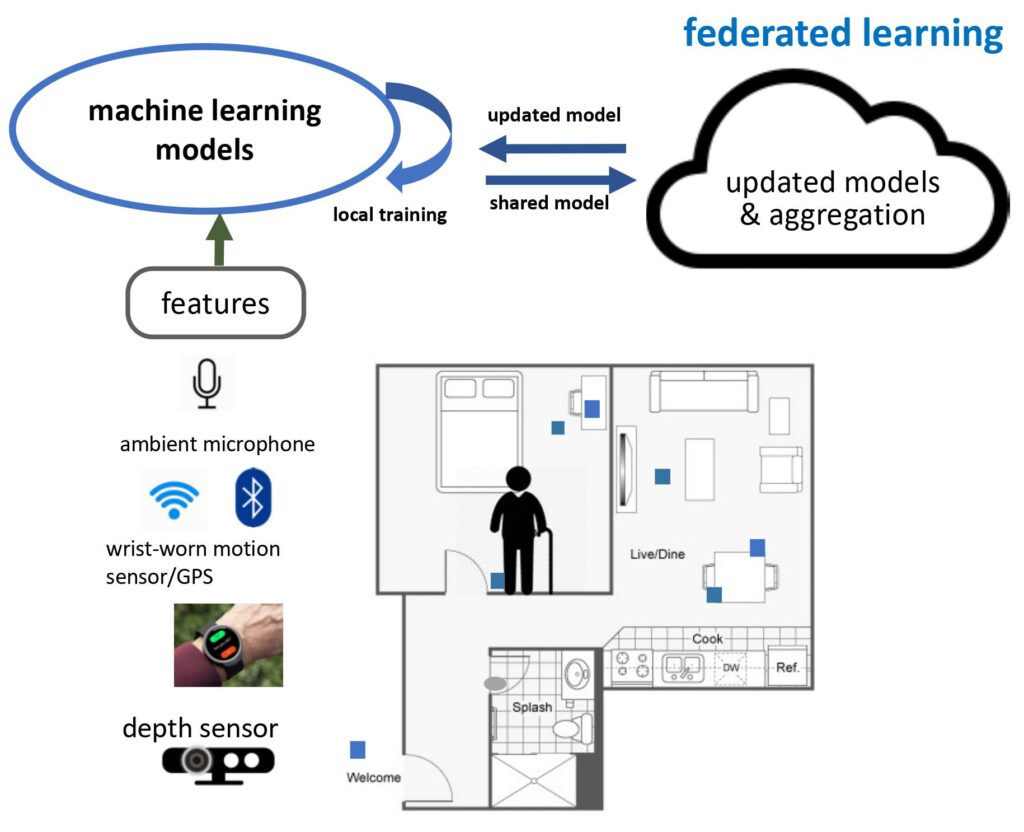
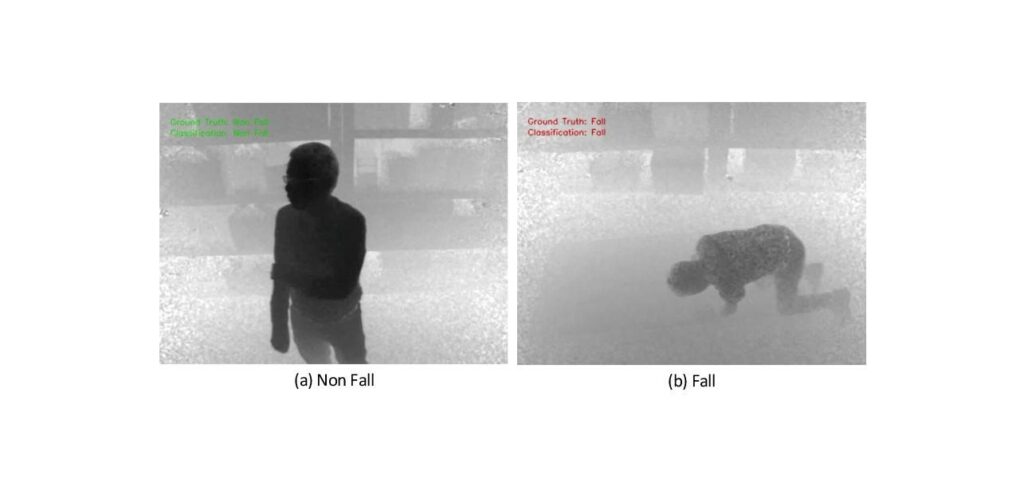
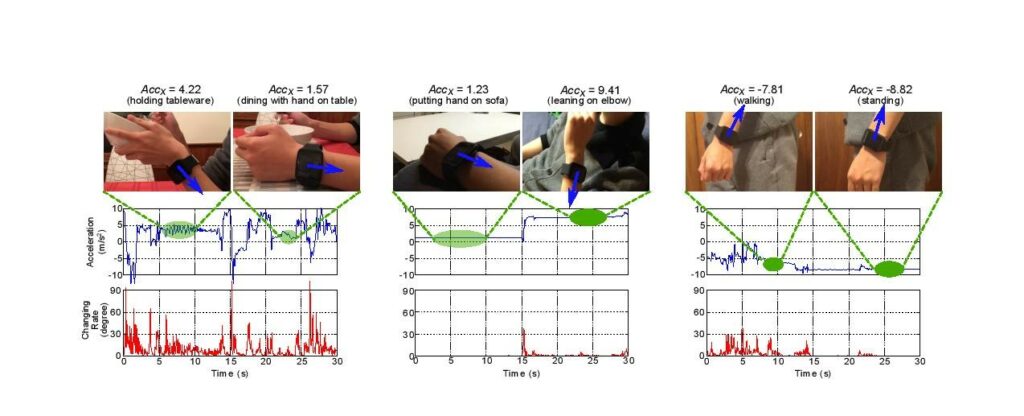
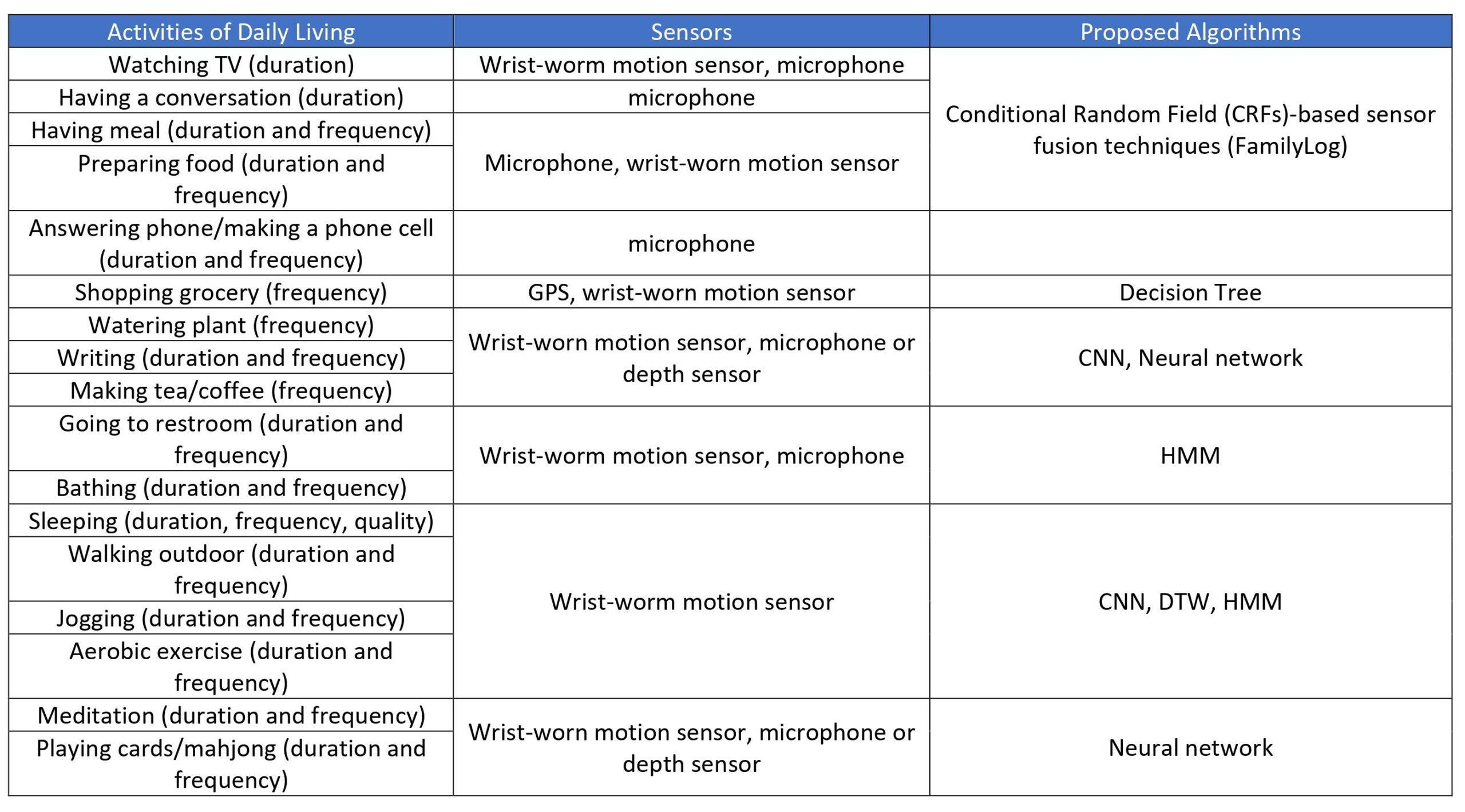
Uniqueness and Competitive Advantages:
- The collected data are stored in the local repositories and are neither being transmitted nor leaked, thus protecting the users’ privacy
- Uses depth cameras to collect depth images of users’ daily activities, which don’t reveal face information
- Provides users with the high-quality early identification of developing AD and timely intervention to slow the progression of AD